A new algorithm to SELECT the best therapy helps advance precision oncology.
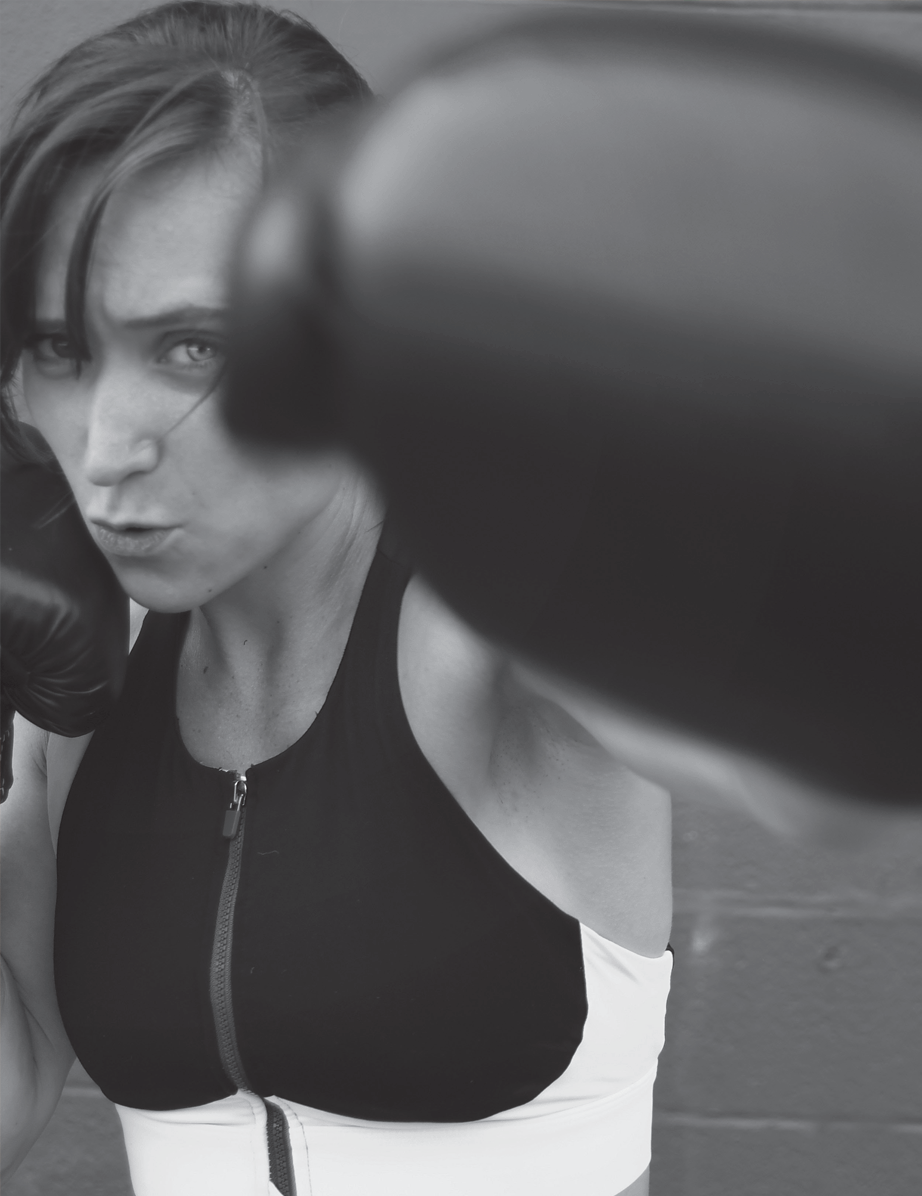
Normal cells can have two pathways for certain essential biological functions in case one fails. In this study, researchers developed a computer algorithm to identify cancer cells that have disabled such backup pathways. By finding cells that already have a mutation in one pathway, the researchers can knock out the second pathway to cause cell death via a “one-two punch.” Credit: Natalie Runnerstrom
Identifying a pathway mutation in a patient’s tumor and using it to guide cancer treatment has become a standard approach to designing personalized cancer treatments. Identifying two pathways that work synergistically may be even better.
A team of computational researchers at CCR have developed a new algorithm, called SELECT, to predict the best treatment strategies for multiple tumor types.1 SELECT uses the powerful concept of synthetic lethality — where the combination of mutations in two pathways packs a one-two punch that results in cell death whereas a mutation in only one pathway alone does not.
The team, led by Senior Investigators Eytan Ruppin, M.D., Ph.D., and Kenneth Aldape, M.D., is using SELECT to identify such pathway pairs and partner them with drugs that can disable a pathway in a cancer cell that already contains a mutation in another. Importantly, SELECT complements current precision oncology approaches that rely on genomic data by analyzing tumor transcriptome information, which represents the complete set of RNA molecules present in a cell and better reflects the cell’s functional status.
The algorithm, described in Cell, also leverages another type of interaction called synthetic rescue, where a pathway mutation reduces the cell’s viability, but a mutation in a different pathway rescues the cell from death. The team is using this information to predict whether checkpoint therapy — a type of immunotherapy — might benefit a patient.
To test SELECT, the researchers used numerous publicly available datasets from targeted, chemotherapy and immunotherapy clinical trials and found that it can predict the efficacy of therapies in individual patients with 80 percent accuracy, outperforming existing prediction models.
Eytan Ruppin, M.D., Ph.D.
Chief
Cancer Data Science Laboratory
Kenneth Aldape, M.D.
Chief
Laboratory of Pathology
Ruppin has been developing prediction algorithms since 2013, and SELECT is his fourth such model. He says that the SELECT algorithm is among the most comprehensive current predictive approaches in oncology but underscores the need for more data to improve the algorithm. “Conceptually, what we are trying to do is actually quite simple. It is the details that are complicated, and sorting them out requires more work and patient data,” he emphasizes.
To obtain enough data for the latest version of SELECT, Ruppin partnered with Aldape with whom he shares a vision of using additional molecular information, such as tumor methylation, proteomics and liquid biopsies, to develop better treatment strategies.
Going forward, with the support of CCR leadership and in collaboration with many clinical colleagues at the NIH Clinical Center, Aldape and Ruppin hope that their tool will stimulate the development of precision oncology-based trials. These trials would prospectively test SELECT’s ability to improve patient treatment and collect more data that could in turn lead to improvements in the underlying SELECT prediction algorithms. Plans are underway to conduct studies in brain, breast, bladder and kidney cancers, among others.
1 SELECT stands for SynthEtic LEthality and rescue-mediated precision onCology via the Transcriptome.