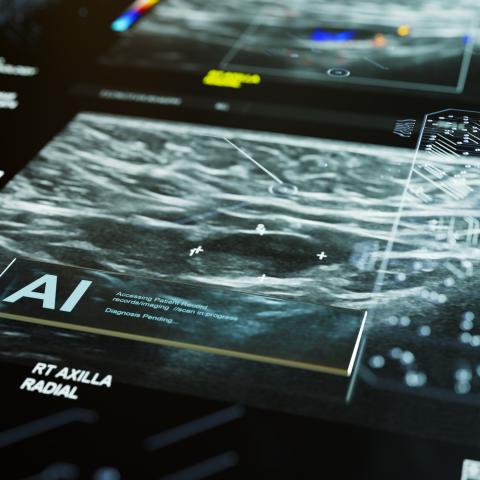
A fictional AI medical bot scanning a patient’s mammogram to diagnose breast cancer. The development of a new AI model, ENLIGHT DEEP-PT, could one day serve a similar purpose. Image: iStock.
Researchers have created a machine-learning algorithm that can analyze images of tumors and recommend individualized treatments. A study published July 3, 2024, in Nature Cancer reports that a novel approach, called ENLIGHT DEEP-PT, sidesteps the need for sequencing analysis of tumor samples and was found to be highly accurate.
If doctors know the genetic subtype of a tumor, they can often recommend more targeted and effective therapies for their patients. However, gaining detailed insights into the genetic signature of a tumor involves time-consuming and expensive sequencing analyses of the patient’s tumor sample.
“Sometimes doctors and patients don’t have that much time — there’s a need to start treatment right away,” explains Eytan Ruppin, M.D., Ph.D., Chief of the Cancer Data Science Laboratory and Head of the Computational Precision Oncology Section. His lab sought to develop a faster approach using recent advances in image analysis via artificial intelligence (AI).
To this end, he partnered with Danh-Tai Hoang, Ph.D., at Australian National University, who trained at NIH, to develop a machine-learning algorithm that could analyze images of tumors — currently used in standard pathology labs for tumor diagnosis — to predict gene expression (activity) based solely on the physical appearance of the tumor. This AI program, which they named DEEP-PT (Deep Pathology for Transcriptomics), sidesteps the need for expensive RNA sequencing to identify which genes are expressed in the tumor.
The predicted gene expression is then fed to a second program called ENLIGHT that can predict which treatment is best suited for a patient based on the DEEP-PT results. ENLIGHT was created in previous work led by a start-up company called Pangea Biomed, the Ruppin lab and other groups at NCI.
The researchers combined the two models to form a single program called ENLIGHT DEEP-PT. They tested its ability to predict the patient’s response on completely new datasets of patient tumor samples that the program had never been trained on or seen. They found its predictive performance to be quite accurate: if ENLIGHT DEEP-PT predicted that a patient would respond to a given therapy, that person would indeed be two to five times more likely to respond than a patient who was predicted not to respond to it. This was true across five different therapies.
“We were very pleasantly surprised,” says Ruppin, noting that further testing of ENLIGHT DEEP-PT is needed before it can be used in clinical practice. However, if proven beneficial in independent prospective clinical trials, it could help many patients receive more individualized and effective therapy in a timely and cost-effective manner.
Of particular importance, tumor sequencing often isn’t an option in developing countries and other underserved regions. But ENLIGHT DEEP-PT could help patients and doctors make more informed treatment decisions without the need for costly sequencing.
“ENLIGHT DEEP-PT analysis can be done in any place,” explains Ruppin, emphasizing how this technology could lead to more widespread precision medicine for everyone. “Just imagine the impact if indeed it is proven beneficial in prospective studies, as we hope.”