New computational tool analyzes gene expression to predict which melanoma patients will benefit from immunotherapy.
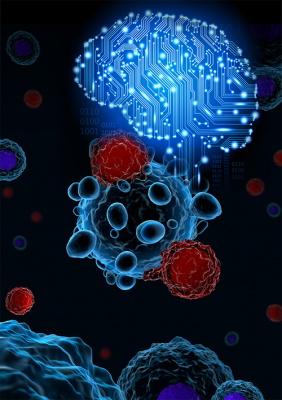
A new predictive computational tool developed by CCR scientists analyzed gene expression in melanoma to determine if the patient’s cancer is likely to respond to immunotherapy. This image shows a melanoma cell being attacked by immune cells. Credit: iStock
Although immunotherapy is radically changing how we treat some cancers, these treatments, which boost the ability of a patient’s own immune system to fight cancer cells, do not work for everyone. Clinicians need better tools to identify which patients are likely to benefit from immunotherapies available today.
A new computational method developed by CCR scientists and published in Nature Medicine may help patients with melanoma and their doctors decide whether to choose a form of immunotherapy that uses immune checkpoint inhibitors, which are effective for some but not all patients with late-stage melanoma and certain other types of cancer.
Led by CCR Senior Investigator Eytan Ruppin, M.D., Ph.D., who heads CCR’s new Cancer Data Science Laboratory, the team developed a predictive computational tool based on a small set of genes whose activity predicts whether a melanoma patient’s cancer is likely to respond to the checkpoint inhibitors pembrolizumab (Keytruda), nivolumab (Opdivo) or ipilimumab (Yervoy). When tested on data from nearly 300 patients, the algorithm correctly identified nearly every patient whose melanoma responded to these drugs and more than half of those who did not.
The success is notable because predictive tools have been difficult to develop, in part because immunotherapy is so new that relatively few patients have received treatments. As a result, there are few datasets that researchers can mine for correlations between the molecular makeup of patients’ tumors, their responses to immunotherapy and other factors that might influence that response.
Lacking a sufficiently large dataset from patients with melanoma treated with immunotherapy, Ruppin’s team began their efforts by searching elsewhere for clues about features that might underlie an effective anticancer immune response. They looked at neuroblastoma, a cancer that starts in immature nerve cells and often regresses on its own in very young patients. This spontaneous regression is thought to be mediated by the immune system. The team’s hope, explains Noam Auslander, Ph.D., a former graduate student in Ruppin’s laboratory and the first author of this study, was that tumor samples from patients whose immune systems were able to control neuroblastoma might share certain defining features with the tumors of patients whose immune systems were, with the support of immunotherapy, capable of effectively attacking melanoma.
Analyzing gene expression data from patients with neuroblastoma, the team identified 15 checkpoint-related pairs of genes whose relative activity could be used to predict the effectiveness of the immune system’s anticancer response, which they used to develop a predictive tool. When they applied this predictor to available data from patients with melanoma, it outperformed all other predictors of immunotherapy drug outcomes that had been reported so far.
The next steps will be to refine and validate the predictor before it can be used clinically and to test whether the same approach can be used to predict immunotherapy responses for patients with other types of cancer. Ultimately, tools like these will be critical in enabling a more personalized approach to cancer immunotherapy.