Modern biomedical research and clinical care generate more data than ever. These records are creating rich datasets, which can be mined with new artificial intelligence and machine learning tools for diagnoses, prediction of treatment outcomes and design of new treatment strategies.
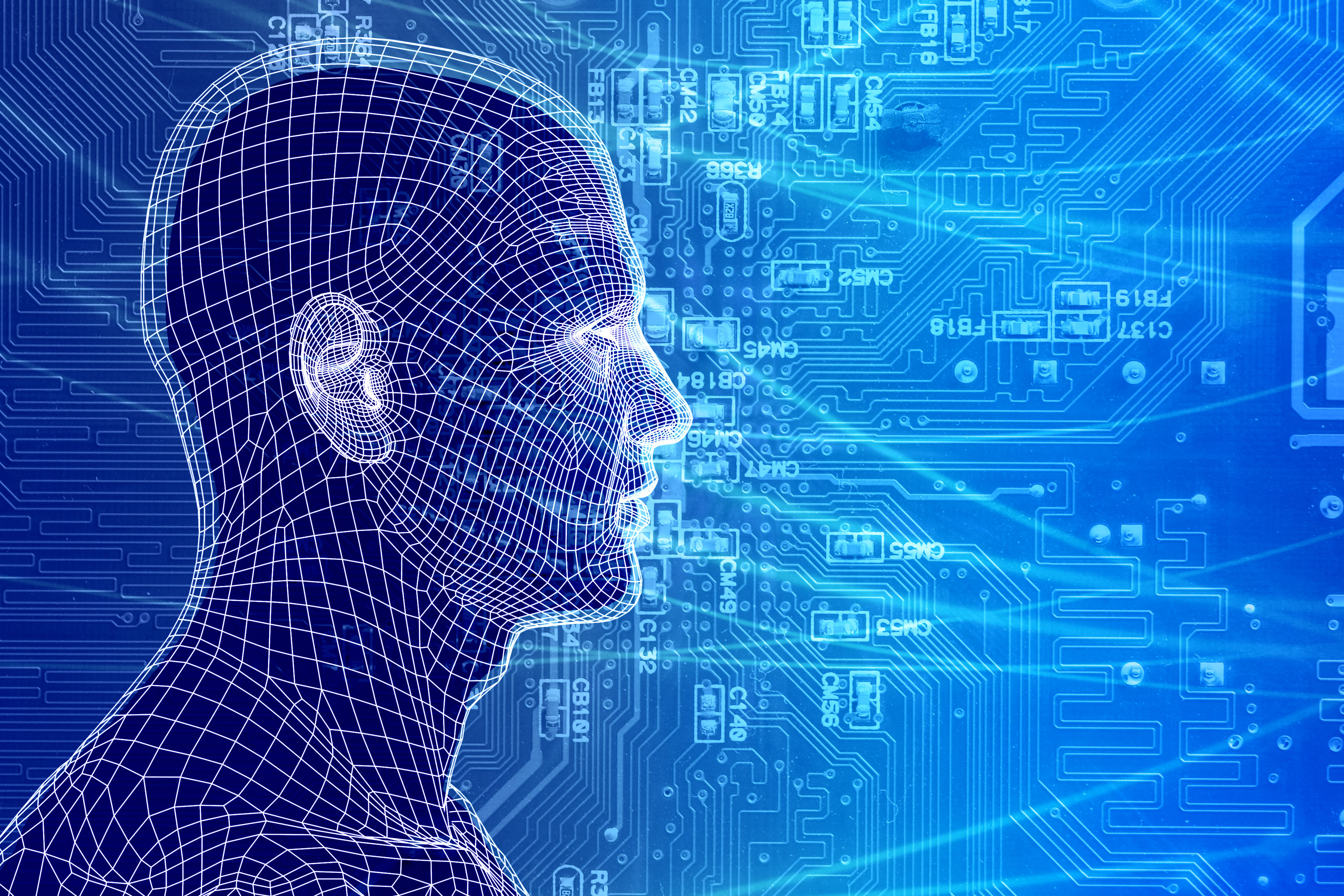
Credit: iStock
Modern biomedical research and clinical care generate more data than ever. These records are creating rich datasets, which can be mined with new artificial intelligence and machine learning tools for diagnoses, prediction of treatment outcomes and design of new treatment strategies.
Data is the foundation of all scientific endeavors. The ability to collect and analyze large datasets provides unprecedented opportunity to understand cancer at every level, from molecular signatures to nationwide health statistics, including health disparities, and to help make treatment decisions based on the knowledge distilled from these massive collections.
Once available only to research institutions with extensive data storage and computing capabilities, these datasets are increasingly available to the wider researcher community through public repositories such as NCI’s Genomic Data Commons, which integrates data from large, collaborative national projects such as The Cancer Genome Atlas and the Therapeutically Applicable Research to Generate Effective Therapies (TARGET) initiative. These repositories provide systems to securely store, share and analyze this information without compromising patient identity.
The availability of public data collections has spurred development of software tools designed to store, process, analyze and visualize large datasets. In turn, a new generation of computational scientists has emerged who apply the latest tools to the analysis of cancer data.
At the forefront of these tools and methodologies are machine learning and artificial intelligence (AI) approaches in which computer networks are programmed to rapidly analyze complex biomedical data and find hidden patterns researchers may not have seen.
For example, in computer vision, software can detect and quantify abnormal features on medical images that may be missed by the human eye, such as subtle patterns of changes in tissues, leading to an earlier or more accurate diagnosis.
A pie chart representing data available in the NCI Genomic Data Commons (GDC) categorized by major site of origin. The GDC contains de-identified data from more than 32,000 patients with more than 60 different types of cancer gathered from 40 different projects. Credit: NCI Genomic Data Commons
Similarly, AI can detect complex changes in gene activity in response to cancer drug treatment. And by comparing these cellular activities with the genetics of a patient, AI can predict the likelihood of treatment success. These methods will ultimately be used to pick the best possible treatment for a patient even before they start their cancer therapy.
Another set of digital technologies is enabling patients themselves to contribute data to clinical trials. Biometric sensors and fitness trackers, as well as health apps on patients’ phones and mobile devices, allow researchers to obtain real-time reports on items such as pain and activity levels to supplement information collected during intermittent clinic visits.
Finally, in the realm of public health, more sophisticated ways to monitor cancer cases and to relate them to environmental, genetic and socioeconomic factors are becoming available.
Big data and AI are already transforming cancer research and cancer care and will become a cornerstone of every aspect of cancer research and treatments.