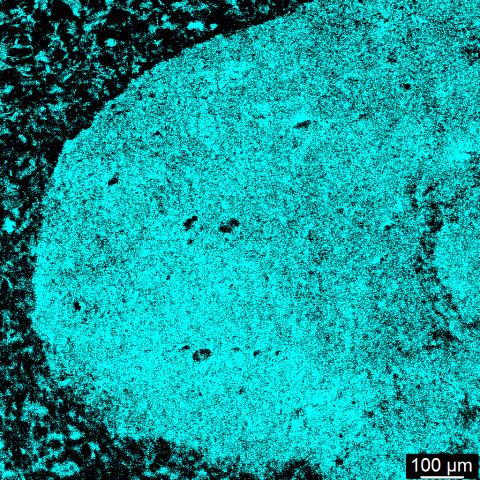
The new AI tool, APOLLO, is able to analyze spectral data of tumors to identify features of interest. In this image, it was used to identify two frequencies important for identifying tumor (blue) tissue compared to non-tumor tissue. Image credit: Larion lab
A new machine learning model is able to sift through spectral data derived from brain tumor tissue to reveal a wealth of information, including gene activations and mutations, and even which lipid molecules are present in the tumors. The new artificial intelligence (AI) model, called rAman-based PathOLogy of maLignant gliOma (APOLLO), is described in a study published online June 3, 2024, in Neuro-Oncology. The result could one day help doctors quickly identify their patient’s tumor subtype and recommend better and more targeted therapies.
Gliomas are a type of brain cancer with a low survival rate, underscoring the need for more knowledge and tools to treat the disease. Mioara Larion, Ph.D., Investigator in the Neuro-Oncology Branch, is interested in gaining a better understanding of the biological differences of various glioma subtypes.
To do so, Larion and her lab partnered with collaborators at the University of Turku, Finland, to create APOLLO, a novel machine learning platform that analyzes spectral data of tumors. First, a laser is directed toward a tissue sample, and then the scattered light is used to create a 3-D matrix of the tumor.
Larion and her colleagues trained their APOLLO model to analyze these detailed, 3D matrices of gliomas and detect various underlying characteristics. Remarkably, the AI could determine whether or not a tumor has a genetic mutation called IDH1 — which is associated with less aggressive disease — with 82% accuracy. As well, APOLLO was able to predict the silencing of tumor genes — which occurs through a process called DNA methylation — with 77% or higher accuracy.
Larion points out that the DNA methylation in IDH1-mutated glioma is strongly associated with the aggressiveness of the tumor. “Because methylation plays such an important role in the outcome of disease, I think APOLLO will be a fast way [for scientists] to classify IDH1-mutated brain tumors as aggressive or indolent,” Larion explains.
Traditional techniques to analyze tissue samples require that the wax used in the preservation technique is removed before the laser can create 3D matrices of the tumors. This process also happens to wash away the lipids embedded in the tumor.
However, APOLLO can analyze tumor samples without washing away the wax and lipids, providing researchers with an unprecedented look at the lipid profiles of tumors as well. Using APOLLO, the researchers were surprised to find that certain lipid profiles — ones with an increased presence of cholesterol molecules with long fatty acid chains — were associated with less aggressive gliomas and lower mortality rates.
Larion notes that APOLLO has already helped uncover new insights into gliomas and that similar AI models could be developed for other cancer types as well. “I think this approach will be very broadly applicable,” she says.
In future work, Larion plans to study these differences in IDH1 mutations and lipid profiles in more detail. She also hopes to make APOLLO available for more researchers to use, so that further progress can be made to understand the inner workings of gliomas.