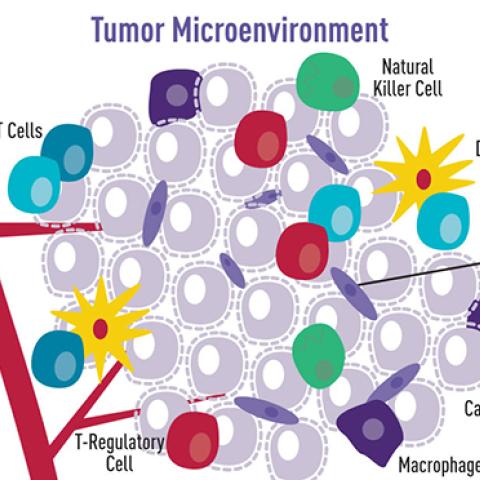
Cartoon illustration showing how a variety of cell types surround and interact with cancer cells. This tumor microenvironment can impact tumor cell behavior.
Image source: NCI Visuals Online
CCR researchers created a new way to process tumor data computationally, giving them the ability to predict the cell-type-specific gene expression in a tumor sample from its bulk expression, in thousands of tumor samples with minimal cost. Led by Eytan Ruppin, M.D., Ph.D., chief of the Cancer Data Science Laboratory, the researchers used their computational approaches called CODEFACS (COnfident DEconvolution For All Cell Subsets) and LIRICS (LIgand-Receptor Interaction between Cell Subsets) to build a set of predictions for response of melanomas to immunotherapy. Their work, reported Jan. 4, 2022, in Cancer Discovery, offers a new approach to characterizing individual tumor cell types from established bulk datasets.
Tumor sequencing initiatives have amassed bulk DNA and RNA data from a mixture of cell types in tumor samples, but single-cell information is still limited, costly and quite scarce. Using a computational process called deconvolution, the team reverse engineered cell-type-specific gene expression data from each tumor sample in The Cancer Genome Atlas. They validated their findings with existing single-cell sequencing, outperforming current deconvolution methods.
“What makes CODEFACS unique is the incorporation of confidence estimates that tell a user how reliable the gene expression prediction is,” says Sushant Patkar, Ph.D., co-first author on the study. “We are taking bulk data and extracting a virtual proxy of the single-cell information. While we don’t get the whole distribution, we still get confidence estimates of the average expression of many genes in each cell type in a given sample, which is a critical part of this method.”
Adding the computational approach of LIRICS, they further characterized the cell-cell communication landscape of each tumor sample. Their work revealed key tumor-immune interactions linked with enhanced response to certain immunotherapies for solid tumors that fail to correct one type of mistake frequently made when DNA is copied in dividing cells, compared to other highly mutated tumors across cancer types. Furthermore, in a set of melanoma tumors, the team predicted patient responses to immunotherapy better than other published methods.
“We can evaluate contacts between tumor cells and the immune system and build a set of interaction details that predict patient response to immunotherapy,” says Kun Wang, Ph.D., co-first author on the study. “These tools can also point researchers to those interactions that are likely to be the most important.”
Ruppin and Alejandro A. Schäffer, Ph.D., a staff scientist in Ruppin’s lab, hope ultimately to classify patients into subgroups based on gene expression profiles of tumor cells and their microenvironments to best match patients to potential treatments. They are already collaborating with Louis M. Staudt, M.D., Ph.D., to classify B-cell lymphoma tumors into response-relevant genomic subtypes using the improved computational methods.