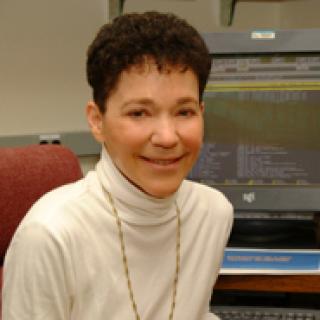
Ruth Nussinov, Ph.D.
- Center for Cancer Research
- National Cancer Institute
- Building 542, Rm 603
- Frederick, MD 21702-1201
- 301-846-5579
- ruth.nussinov@nih.gov
RESEARCH SUMMARY
Dr. Nussinov is a biologist by training. Her dissertation proposed the dynamic programming algorithm for predicting the secondary structure of single stranded RNA. It is still the leading method for RNA folding to this date.
Her most important discovery was in the 1990s. In 1999 Nussinov published the transformational concept that all conformations preexist—even if the crystal captures only one—and that evolution harnesses their dynamic interconversion for function, dispelling the dogma that only the wild-type shape is relevant. Nussinov suggested a vastly different scenario from the-then dogma of two, “open” and “closed” conformations proposed by Monod, Wyman, and Changeux. She proposed that there is not one folded form, nor two—as they suggested—but many different forms, and in equilibrium, the system keeps jumping between them, and that this barrier-crossing is function. The concept that she suggested is significant since it explained that rather than the ligand inducing a conformational change (as in induced fit), the ligand can select a preexisting (relatively rare, non-minimal energy) conformation in the system that may be better suited to dock it, with minor optimization. It will then bind the ligand, and the equilibrium will keep producing more of this conformation to compensate, which she suggested (also in 1999), is the allosteric effect. This foundational “conformational selection and population shift” idea as an alternative to the “induced fit” text-book model explains the mechanism of molecular recognition. The dynamic shifts among conformations explain catalysis (2000), regulation, kinase activation, and allosteric drugs actions. Her concept was confirmed by innumerable experiments and is now widely established. As Nussinov and others have shown since, this paradigm helps unravel diverse processes as signaling, regulation, and aggregation in amyloid diseases, and oncogenic transformation, contributing to extraordinary advancements in understanding structure and function. Her pioneering work has contributed to extraordinary advances in understanding the conformational behaviors of biological macromolecules, their uncontrolled actions in disease, and form the basis for allosteric drug actions. For her contributions to the broad area of molecular recognition and allostery, she has been recognized as Pioneer in Molecular Biology. https://www.sciencedirect.com/science/article/pii/S002228362500110X?via%3Dihub#f0015
Dr. Nussinov served as the Editor-in-Chief of PLOS Computational Biology and is currently Editor-in-Chief of Current Opinion in Structural Biology. She is an elected Fellow of the Biophysical Society, the International Society of Computational Biology (ISCB), the American Physical Society (APS), the American Institute for Medical and Biological Engineering (AIMBE), and EMBO Fellow. She was recognized by multiple Domestic and International Awards. She has been designated multiple times a Highly Cited Researcher (ranking among the top 3000 researchers or 1% across all fields according to Thomson Reuters Essential Science Indicators, http://highlycited.com/ "earning them the mark of exceptional impact"). and her impact among the top female scientists was ranked as 44 in the US (66 world-wide), https://research.com/scientists-rankings/best-female-scientists. Most of her publications are conceptualized and driven by her group.
Recently, we developed KDS (Kinase Drug Selectivity) software for the human kinome, https://github.com/CBIIT/KDS.
A personal scientific overview of her biography has been published in
http://www.sciencedirect.com/science/article/pii/S0165614717301293?via%3Dihub Trends Pharmacol Sci. 2017 Sep;38(9):761-763. doi: 10.1016/j.tips.2017.06.008.
Autobiography of Ruth Nussinov.
Nussinov R. J Phys Chem B. 2021 Jul 1;125(25):6735-6739. doi:10.1021/acs.jpcb.1c04719
Her scientific biography highlighting her accomplishments has been published in 2025 as Pioneer in Molecular Biology. https://www.sciencedirect.com/science/article/pii/S002228362500110X?via%3Dihub#f0015
Areas of Expertise
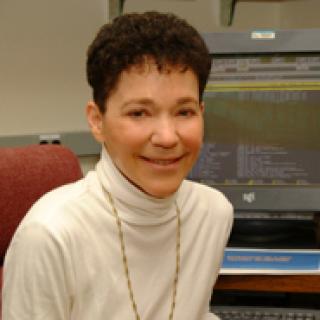
Ruth Nussinov, Ph.D.
Research
An Overview of Our On-Going Research
We are a theoretical/computational group. Our work distinguishes itself by its conceptual depth, breadth, and innovative view. We tackle significant biological and translational questions.
Primary among these is cancer initiation, drug resistance and cell proliferation. Recently, we expanded our focus to include neurodevelopmental disorders and cancer. We took up puzzling questions such as How can same-gene mutations promote both cancer and developmental disorders? (Nussinov R, Tsai CJ, Jang H. Sci. Adv. 2022 Jan 14;8(2):eabm2059. doi: 10.1126/sciadv.abm2059), and why those with neurodevelopmental disorders have a high risk of cancer. We further proposed that Neurodevelopmental disorders, immunity, and cancer are connected (Nussinov R, Tsai CJ, Jang H. iScience. 2022 May 30;25(6):104492. doi: 10.1016/j.isci.2022.104492).
Recent examples of the significant conceptual questions that we undertook include:
- Anticancer drug resistance: An update and perspective (Nussinov R, Tsai CJ, Jang H. Drug Resist. Updat. 2021 Dec;59:100796. doi: 10.1016/j.drup.2021.100796). The dilemma that the clinical oncologist is facing is that not all the genomic alterations as well as alterations in the tumor microenvironment that facilitate cancer cell proliferation are known, and neither are the alterations that are likely to promote metastasis. For example, the common K-RasG12C driver mutation emerges in different cancers. Most occur in NSCLC, but some occur, albeit to a lower extent, in colorectal cancer and pancreatic ductal carcinoma. The responses to K-RasG12C inhibitors are variable and fall into three categories, (i) new point mutations in K-Ras, or multiple copies of KRAS G12C which lead to higher expression level of the mutant protein; (ii) mutations in genes other than KRAS; (iii) original cancer transitioning to other cancer(s). Here we discuss the molecular mechanisms underlying drug resistance while focusing on those emerging to common targeted cancer drivers. We also address questions of why cancers with a common driver mutation are unlikely to evolve a common drug resistance mechanism, and whether one can predict the likely mechanisms that the tumor cell may develop. These vastly important and tantalizing questions in drug discovery, and broadly in precision medicine, are the focus of our present review.
- Allostery, and how to define and measure signal transduction (Nussinov R, Tsai CJ, Jang H. Biophys. Chem. 2022 Apr;283:106766. doi: 10.1016/j.bpc.2022.106766). Here we ask: What is productive signaling? How to define it, how to measure it, and most of all, what are the parameters that determine it? Further, what determines the strength of signaling from an upstream to a downstream node in a specific cell? These questions have either not been considered or not entirely resolved. The requirements for the signal to propagate downstream to activate (repress) transcription have not been considered either. Yet, the questions are pivotal to clarify, especially in diseases such as cancer where determination of signal propagation can point to cell proliferation and to emerging drug resistance, and to neurodevelopmental disorders, such as RASopathy, autism, attention-deficit/hyperactivity disorder (ADHD), and cerebral palsy.
- Allostery: Allosteric Cancer Drivers and Innovative Allosteric Drugs (Nussinov R, Zhang M, Maloney R, Liu Y, Tsai CJ, Jang H. J. Mol. Biol. 2022 Apr;167569. doi: 10.1016/j.jmb.2022.167569). Here, we discuss the principles of allosteric activating mutations, propagation downstream of the signals that they prompt, and allosteric drugs, with examples from the Ras signaling network. We focus on Abl kinase where mutations shift the landscape toward the active, imatinib binding-incompetent conformation, likely resulting in the high affinity ATP outcompeting drug binding.
- Mechanism of activation and the rewired network: New drug design concepts (Nussinov R, Zhang M, Maloney R, Tsai CJ, Yavuz BR, Tuncbag N, Jang H. Med. Res. Rev. 2022 Mar;42(2):770-799. doi: 10.1002/med.21863). Precision oncology benefits from effective early phase drug discovery decisions. Recently, drugging inactive protein conformations has shown impressive successes, raising the cardinal questions of which targets can profit and what are the principles of the active/inactive protein pharmacology. Our structure-based review and outlook updates the traditional Mechanism of Action, informs decisions, and calls attention to the intrinsic activation mechanism of the target protein and the rewired tumor-specific network.
- Inhibition of Nonfunctional Ras (Nussinov R, Jang H, Gursoy A, Keskin O, Gaponenko V. Cell Chem. Biol. 2021 Feb 18;28(2):121-133. doi: 10.1016/j.chembiol.2020.12.012). Intuitively, functional states should be targeted, not nonfunctional ones. So why could drugging the inactive K-Ras4BG12C work -but drugging the inactive kinase will likely not? Collectively, here we postulate that drug action relates to blocking the mechanism of activation, not to whether the protein is in the active or inactive state.
Examples of recent significant questions that can be addressed by structural computations that we took up include:
- The mechanism of activation of MEK1 by B-Raf and KSR1 (Maloney RC, Zhang M, Liu Y, Jang H, Nussinov R. Cell Mol. Life Sci. 2022 May 4;79(5):281. doi: 10.1007/s00018-022-04296-0). MEK1 interactions with B-Raf and KSR1 are key steps in Ras/Raf/MEK/ERK signaling. Despite this, vital mechanistic details of how these execute signal transduction are still enigmatic. Among these is why, despite B-Raf and KSR1 kinase domains similarity, the B-Raf/MEK1 and KSR1/MEK1 complexes have distinct contributions to MEK1 activation, and broadly, what is KSR1's role.
- The mechanism of Raf activation through dimerization (Zhang M, Maloney R, Jang H, Nussinov R. Chem. Sci. 2021 Nov 18;12(47):15609-15619. doi: 10.1039/d1sc03444h). Despite its clinical importance, fundamental questions, such as how the side-to-side dimerization promotes the OFF-to-ON transition of Raf's kinase domain and how the fully activated ON-state kinase domain is stabilized in the dimer for Raf signaling, remain unanswered. This work provides atomic level insight into critical steps in Raf activation and outlines a new venue for drug discovery against Raf dimerization.
- The mechanism of full activation of tumor suppressor PTEN at the phosphoinositide-enriched membrane (Jang H, Smith IN, Eng C, Nussinov R. iScience. 2021 Apr 17;24(5):102438. doi: 10.1016/j.isci.2021.102438). Tumor suppressor PTEN, the second most highly mutated protein in cancer, dephosphorylates signaling lipid PIP3 produced by PI3Ks. Excess PIP3 promotes cell proliferation. The mechanism at the membrane of this pivotal phosphatase is unknown hindering drug discovery.
- The mechanism of PI3Kα activation at the atomic level (Zhang M, Jang H, Nussinov R. Chem. Sci. 2019 Feb 20;10(12):3671-3680. doi: 10.1039/c8sc04498h). PI3K lipid kinases phosphorylate PIP2 to PIP3 in the PI3K/Akt/mTOR pathway to regulate cellular processes. They are frequently mutated in cancer. Here we determine the PI3Kα activation mechanism at the atomic level.
An Overview of Our Earlier Research
Conformational Selection and Population Shift. Inspired by the milestone concept of the free energy landscape (1991), we proposed in 1999 the model of “conformational selection and population shift” as an alternative to the “induced fit” model to explain molecular recognition. According to the “induced fit” hypothesis, the initial interaction between a protein and a binding partner induces a conformational change in the protein through a stepwise process. In our “conformational selection” model it is assumed that, prior to binding, the unliganded protein exists as an ensemble of all possible conformations in dynamic equilibrium. The binding partner interacts preferentially with a weakly populated, higher-energy conformation, causing the equilibrium to shift in favor of the selected conformation. This conformation then becomes the major conformation in the complex. Importantly, there cannot be a conformational selection without a population shift. As we explained then, population shift, or the redistribution of the pre-existing conformational ensemble of the macromolecule, is the origin of allostery. A recent paper in Science noted, “Although biochemistry textbooks have championed the induced fit mechanism for more than 50 years, there is now growing support for the additional [conformational selection and population shift] binding mechanism” (quoted from Boehr DD and Wright PE. How do proteins interact? Science 320: 1429-30, 2008).
Conformational selection has by now been observed for protein-ligand, protein-protein, protein-DNA, protein-RNA and RNA-ligand interactions. These data support the powerful new molecular recognition paradigm for processes as diverse as signaling, catalysis, gene regulation, and protein aggregation in disease. The “conformational selection and population shift” model has significantly impacted views and strategies in allosteric drug discovery, biomolecular engineering and molecular evolution. Some early references for our proposed model:
- Folding funnels, binding funnels, and protein function. Tsai CJ, Kumar S, Ma B, Nussinov R. Protein Sci. 8: 1181-90, 1999.
- Folding funnels and binding mechanisms. Ma B, Kumar S, Tsai CJ, Nussinov R. Protein Eng. 12: 713-20, 1999.
- Folding and binding cascades: shifts in energy landscapes. Tsai CJ, Ma B, Nussinov R. Proc .Natl. Acad. Sci.USA. 96: 9970-72, 1999.
- Folding and binding cascades: dynamic landscapes and population shifts. Kumar S, Ma B, Tsai CJ, Sinha N, Nussinov R. Protein Sci. 9: 10-9, 2000.
- Multiple diverse ligands binding at a single protein site: a matter of pre-existing populations. Ma B, Shatsky M, Wolfson HJ, Nussinov R. Protein Sci. 11: 184-97, 2002.
We further proposed conformational selection and population shift in protein disorder (Structured disorder and conformational selection. Tsai CJ, Ma B, Sham YY, Kumar S, Nussinov R. Proteins 44: 418-27, 2001).
In 1999 we also proposed that the energy landscape and the “conformational selection and population shift” model are related to protein function: “Here we extend the utility of the concept of folding funnels, relating them to biological mechanisms and function..." (Ref. 1). “Whereas previously we have successfully utilized the folding funnels concept to rationalize binding mechanisms... here we further extend the concept of folding funnels, illustrating its utility in explaining enzyme pathways, multimolecular associations, and allostery. This extension is based on the recognition that funnels are not stationary; rather, they are dynamic, depending on the physical or binding conditions.” (Ref. 4). We suggested that the energy landscape and the minima at the bottom of the funnel explain multiple binding events in signaling (Ref. 3): “For each such [binding] event, the population around the bottom of the corresponding (folding or binding) funnel serves as the repertoire of potentially available molecules for the following binding event in the chain. As in the case of the conformers present around the bottom of the folding funnel, here, too, it is not the conformer with the highest population times that will bind in the following step. Rather, it is the conformer whose structure in the current bound stage is most favorable for the next binding event.”
In 2004 we suggested that all proteins are allosteric (Is allostery an intrinsic property of all dynamic proteins? Gunasekaran K, Ma B, Nussinov R. Proteins 57: 433-43, 2004) and, more recently, we provided the unified mechanistic underpinnings of allostery (A unified view of "how allostery works". Tsai CJ, Nussinov R. PLoS Comput Biol. 10: e1003394, 2014), the underappreciated role of allostery in the cell (The underappreciated role of allostery in the cellular network. Nussinov R, Tsai CJ, Ma B. Annu Rev Biophys. 42: 169-89, 2013), the principles of allosteric interactions in cell signaling (Principles of allosteric interactions in cell signaling. Nussinov R, Tsai CJ, Liu J. J Am Chem Soc. 136(51): 17692-701, 2014), its role in disease (Allostery in disease and in drug discovery. Nussinov R, Tsai CJ. Cell 153: 293-305, 2013), and, over the years, the design principles of allosteric drugs (e.g., Unraveling structural mechanisms of allosteric drug action. Nussinov R, Tsai CJ. Trends Pharmacol Sci. 35: 256-64, 2014; The design of covalent allosteric drugs. Nussinov R, Tsai CJ. Annu Rev Pharmacol Toxicol. 55: 249-67 2014).
Preexisting conformational ensembles in catalysis. By 2000 we had also extended our pre-existing ensembles view to enzyme catalysis: “The widely accepted view of enzymatic catalysis holds that there is tight binding of the substrate to the transition-state structure, lowering the activation energy. This picture may, however, be oversimplified. The real meaning of a transition state is a surface, not a single saddle point on the potential energy surface. … This view of the transition-state ensemble is consistent with the nature of the protein molecule, as embodied and depicted in the protein energy landscape of folding and binding funnels.” (Transition-state ensemble in enzyme catalysis: possibility, reality, or necessity? Ma B, Kumar S, Tsai CJ, Hu Z, Nussinov R. J Theor Biol. 203: 383-97, 2000). More recently, we argued that conformational transitions may involve conformational selection and induced fit, which can be viewed as a special case in the catalytic network (Enzyme dynamics point to stepwise conformational selection in catalysis. Ma B, Nussinov R. Curr Opin Chem Biol. 14: 652-9, 2010).
Protein-protein interactions. We are interested in protein-protein interactions. We proposed that protein folding and binding are similar processes with similar underlying mechanisms, and that the architectural motifs at protein-protein interfaces resemble those of single chain proteins (Protein-protein interfaces: architectures and interactions in protein-protein interfaces and in protein cores. Their similarities and differences. Tsai CJ, Lin SL, Wolfson HJ, Nussinov R. Crit Rev Biochem Mol Biol. 31: 127-52, 1996). This conceptual similarity, now universally accepted, led us to develop and apply similar biophysical and algorithmic approaches. In particular, it led us to propose in the 1990s and again around 2005 that interface structural similarity exists not only between homologous protein pairs; different proteins can also interact via similar interface architectures and these architectures are similar to those observed in single chain proteins. This recognition inspired the idea that known three-dimensional (3D) interface architectures can be used as templates to identify interacting protein pairs independent of homology or global fold similarity. This has proven to be a powerful strategy, which is now followed for large-scale docking by a number of research groups.
Ras and its major pathways, MAPK and PI3K/PTEN/AKT/PDK1/mTOR in tumor proliferation. We took up the challenging problem of the mechanisms of the Ras protein, particularly focusing on K-Ras4B-driven cancers. We addressed key questions, including Ras regulation at the membrane, signaling through its major (MAPK and PI3K/AKT) pathways, mechanisms of its oncogenic mutations, inhibition, and more. Examples include - Does Ras’ disordered hypervariable region (HVR) have a role beyond membrane anchoring? Does Ras dimerize? If so, why, and how? How is Ras regulated at the membrane and what are the mechanisms of Ras signaling at the membrane? What is the dynamical organization of Ras/Raf assemblies at the membrane? Within this framework, we further took up the critical, though overlooked, role of calmodulin in KRAS-driven adenocarcinomas (The Key Role of Calmodulin in KRAS-Driven Adenocarcinomas. Nussinov R, Muratcioglu S, Tsai CJ, Jang H, Gursoy A, Keskin O. Mol Cancer Res. 13(9): 1265-73, 2015), for which we won an award.
Our 2015 Mol Can Res paper won an Award
Best of AACR journals
We also proposed a new view of Ras isoforms in cancers (A New View of Ras Isoforms in Cancers. Nussinov R, Tsai C-J, Chakrabarti M, Jang H. Cancer Res. 2016 Jan 1;76(1):18-23); that GTP Binding and Oncogenic Mutations May Attenuate Hypervariable Region (HVR)-Catalytic Domain Interactions in Small GTPase KRAS4B, Exposing the Effector Binding Site (Lu S, Banerjee A, Jang H, Zhang J, Gaponenko V, Nussinov R. J. Biol. Chem. 290:28887, 2015) and Mechanisms of Membrane Binding of Small GTPase K-Ras4B Farnesylated Hypervariable Region (Jang H, Abraham SJ, Chavan TS, Hitchinson B, Khavrutskii L, Tarasova NI, Nussinov R, Gaponenko V. J. Biol. Chem. 290:9465, 2015). We revealed the structural basis of oncogenic mutations in K-Ras4B (The Structural Basis of Oncogenic Mutations G12, G13 and Q61 in Small GTPase K-Ras4B. Lu S, Jang H, Nussinov R, Zhang J. Sci. Rep. 6:21949, 2016). We also showed that in principle PDEδ should be able to bind and translocate all Ras isoforms, not only K-Ras4B. Earlier data suggested that PDEδ extracts K-Ras4B and N-Ras from the plasma membrane (PM); but surprisingly not K-Ras4A (PDEδ Binding to Ras Isoforms Provides a Route to Proper Membrane Localization. Muratcioglu S, Jang H, Gursoy A, Keskin O, Nussinov R. J. Phys. Chem. B. 121:5917, 2017). Earlier analysis of the crystal structures advanced that the presence of large/charged residues adjacent to the farnesylated site precludes the PDEδ interaction. We showed that PDEδ can bind to farnesylated K-Ras4A and N-Ras like K-Ras4B - albeit not as strongly. We explained that the weaker binding to PDEδ, coupled with stronger anchoring of K-Ras4A in the membrane (but not of electrostatically neutral N-Ras), can explain the observation of why PDEδ is unable to effectively extract K-Ras4A. We thus proposed that farnesylated Ras isoforms can bind PDEδ to fulfil the required PM enrichment, and argued that the different environments - PM versus solution - can resolve apparent puzzling Ras observations. These are novel insights that would not be expected based on the crystal structures alone provide an elegant rationale for previously puzzling observations of the differential effects of PDEδ on farnesylated Ras family proteins.
Autoinhibition Autoinhibition in Ras effectors Raf, PI3Kα, and RASSF5: a comprehensive review underscoring the challenges in pharmacological intervention. Nussinov R, Zhang M, Tsai CJ, Liao TJ, Fushman D, Jang H. Biophys. Rev. 10:1263, 2018). We determined the autoinhibition and activation mechanisms of PI3K (PI3K Driver Mutations: A Biophysical Membrane-Centric Perspective. Zhang M, Jang H, Nussinov R. Cancer Res. 81:237, 2021; Structural Features that Distinguish Inactive and Active PI3K Lipid Kinases. Zhang M, Jang H, Nussinov R. J. Mol. Biol. 432:5849, 2020; The structural basis for Ras activation of PI3Kα lipid kinase. Zhang M, Jang H, Nussinov R. Phys. Chem. Chem. Phys. 21:12021, 2019; The mechanism of PI3Kα activation at the atomic level. Zhang M, Jang H, Nussinov R. Chem. Sci. 10:3671, 2019), the autoinhibition and activation of B-Raf (The mechanism of Raf activation through dimerization. Zhang M, Maloney R, Jang H, Nussinov R. Chem. Sci. 12:15609, 2021; B-Raf autoinhibition in the presence and absence of 14-3-3. Zhang M, Jang H, Li Z, Sacks DB, Nussinov R. Structure. 29:768, 2021), activation by the V600E mutant (The mechanism of activation of monomeric B-Raf V600E. Maloney RC, Zhang M, Jang H, Nussinov R. Comput. Struct. Biotechnol. J. 19:3349, 2021), the Ras-Raf assembly (The quaternary assembly of KRas4B with Raf-1 at the membrane. Jang H, Zhang M, Nussinov R. Comput. Struct. Biotechnol. J. 18:737, 2020), the activation mechanism of PTEN (The mechanism of full activation of tumor suppressor PTEN at the phosphoinositide-enriched membrane. Jang H, Smith IN, Eng C, Nussinov R. iScience. 24:102438, 2021), the role of KSR1 (The mechanism of activation of MEK1 by B-Raf and KSR1. Maloney RC, Zhang M, Liu Y, Jang H, Nussinov R. Cell Mol. Life Sci. 79:281, 2022), and more, all within the conformational framework that guides our work. We further proposed that some phosphorylation sites are ‘passenger’ sites (Phosphorylation and Driver Mutations in PI3Kα and PTEN Autoinhibition. Nussinov R, Zhang M, Tsai CJ, Jang H. Mol Cancer Res. 19:543, 2021), and more.
An innovative view of the allosteric pharmacology, from the conformational standpoint (Allostery: Allosteric Cancer Drivers and Innovative Allosteric Drugs. Nussinov R, Zhang M, Maloney R, Liu Y, Tsai CJ, Jang H. J. Mol. Biol. doi: 10.1016/j.jmb.2022.167569, 2022; Inhibition of Nonfunctional Ras. Nussinov R, Jang H, Gursoy A, Keskin O, Gaponenko V. Cell Chem. Biol. 28:121, 2021).
Ras nanoclusters. We asked Is Nanoclustering essential for all oncogenic KRas pathways? Can it explain why wild-type KRas can inhibit its oncogenic variant? Nussinov R, Tsai CJ, Jang H. Semin Cancer Biol. 54:114, 2019). We proposed that nanoclustering (or dimerization, or oligomerization) is needed only for the Ras-driven MAPK pathway, but not the PI3K/AKT/mTOR, and that nanoclustering can explain why wild-type K-Ras can inhibit its oncogenic variant. Thus, is nanoclustering essential for all oncogenic K-Ras pathways? We proposed that dimerization (nanoclustering) is needed only for activation of Raf/MAPK - but not PI3K. We explained that Raf’s activation requires dimerization of the catalytic domain. Thus, Raf’s activation requires Ras spatial proximity (whether as dimers oligomers, or nanoclusters). In contract, a monomeric Ras activates PI3K. And why does wild-type Ras inhibit its oncogenic variants? And why K-Ras more so than N-Ras?
Early on, it was observed that wild-type human H-Ras gene can act as an onco-suppressor. Subsequent work revealed that in RAS-mutant cancers, wild-type Ras proteins can act as cancer suppressors, notably when the mutant and the wild-type are the same isoform. Shortly thereafter, it transpired that this is particularly the case for K-Ras. Wild-type K-Ras inhibited colony formation and tumor development in cell lines containing an actively expressed KRAS allele. Subsequent experiments confirmed and expanded on this apparent puzzling observation. Even though it was also shown to hold for other Ras isoforms, such as N-Ras, the trend was not as strong. How to explain these observations, coupled with potential pharmacological implications, fueled experiments searching for answers.
Thus, how then to explain the roles of wild-type Ras proteins with respect to their corresponding oncogenic mutants in human cancers? How can the wild-type K-Ras isoform act as a tumor suppressor in mutant KRAS-driven cancers? Why are the observations particularly robust for K-Ras, rather than N-Ras? And why a wild-type Ras isoform not suppress a mutant isoform of a different type? Further complicating the issue were observations supporting that the wild-type can promote tumorigenesis when the mutant and the wild-type belong to different isoforms, or that neither suppresses nor promotes cancer. In the paper above, we offer a simple clustering-centric interpretation, and a way to test it.
We further reviewed factors influencing Ras lateral diffusion, asking whether oncogenic Ras diffusion speed in the membrane is important for signaling response times and whether it affects ubiquitously all pathways. We suggested that if Ras expression is sufficiently high to dimerize (or form nanoclusters), signaling response of those pathways where dimers (or nanoclusters) are involved corresponds to the speed with which Ras molecules travel in the membrane. On average, the faster the rate at which Ras travels to dimerize, the shorter the time to MAPK signaling; but not PI3Kα. However, we argued that K-Ras speed may not play an important functional role because changes in mobility at this scale are unlikely to be significant. In line with this, despite the anchors' variability, lateral diffusion speeds of K-Ras and H-Ras are similar, as is that of Lck kinase; however, even though with similar anchor, Cdc42 mobility presents a different pattern, commensurate with its role in the positioning of the apical domain, suggesting that mobility evolved for function (Oncogenic KRas mobility in the membrane and signaling response. Semin Cancer Biol. 54:109, 2019).
Finally, we took up the Rap1 puzzle (The Mystery of Rap1 Suppression of Oncogenic Ras. Nussinov R, Jang H, Zhang M, Tsai CJ, Sablina AA. Trends Cancer. 6:369, 2020). Decades ago, Rap1, a small GTPase very similar to Ras, was observed to suppress oncogenic Ras phenotype, reverting its transformation. The proposed reason, persisting since, has been competition between Ras and Rap1 for a common target. Yet, none was found. There was also Rap1’s puzzling suppression of Raf-1 versus activation of B-Raf. Reemerging interest in Rap1 envisages capturing its Ras suppression action by inhibitors. Here, we review the literature and resolve the enigma. In vivo oncogenic Ras exists in isoform-distinct nanoclusters. The presence of Rap1 within the nanoclusters reduces the number of the clustered oncogenic Ras molecules, thus suppressing Raf-1 activation and MAPK signaling. Nanoclustering suggests that Rap1 suppression is Ras isoform dependent. Altogether, a potent Rap1-like inhibitor appears unlikely.
Predicting host-pathogen interactions on the structural level. We further proposed host-pathogen interactions (Pathogen mimicry of host protein-protein interfaces modulates immunity. Guven-Maiorov E, Tsai CJ, Ma B, Nussinov R. Semin Cell Dev Biol. 58:136, 2016) and have developed a structural, interface-based method to predict host-pathogen interactions (Prediction of Host-Pathogen Interactions for Helicobacter pylori by Interface Mimicry and Implications to Gastric Cancer. Guven-Maiorov E, Tsai CJ, Ma B, Nussinov R. J Mol Biol. 8:429, 2017). We applied it to all human oncoviruses and constructed a network of the ‘superorganism’ interactions. Recently, we further developed and optimized the method to predict human-microbiome protein-protein interactions focusing on crosstalk between host and microbial proteins (HMI-PRED: A Web Server for Structural Prediction of Host-Microbe Interactions Based on Interface Mimicry. Guven-Maiorov E, Hakouz A, Valjevac S, Keskin O, Tsai CJ, Gursoy A, Nussinov R. J Mol Biol, 432:3395, 2020). We aim to predict which proteins can interact and how, through a structure-based interface mimicry strategy. Efficient and reliable prediction of new interactions can allow identification of potential targets. Powerful protein-protein interaction prediction tools can map interactions and predict how pathogens can hijack signaling in the host cell, which can be tested by experiment. Available experimental structural data are scant, and the combinatorial landscape of host protein-pathogen interactions is vast. We are working to further enhance our server to include more interactions and modeled proteins with AI-adopted prediction methods.
Publications
Molecular principles underlying aggressive cancers
Biography
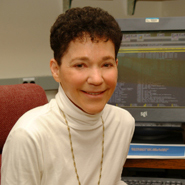
Ruth Nussinov, Ph.D.
Dr. Ruth Nussinov is a senior investigator in the Laboratory of Cancer Immunometabolism, CCR, and a professor emeritus in the Department of Human Genetics, School of Medicine, Tel Aviv University, Tel Aviv, Israel. She received her B.Sc. in microbiology from the University of Washington (Seattle, WA) and her Ph.D. in biochemistry from Rutgers University (New Brunswick, NJ). Her postdoctoral training included a fellowship at the Weizmann Institute, and two visiting scientist positions, one in the Chemistry Department at the University of California, Berkeley, and the other in the Biochemistry Department at Harvard University. Dr. Nussinov then joined the Medical School at Tel Aviv University in 1985 as an associate professor and in 1990 became a full professor. Her association with the NIH started in 1983, first with the National Institute of Child Health and Human Development and, since 1985, with the National Cancer Institute. At Tel Aviv University Dr. Nussinov directed a large group of graduate students at the Medical and Computer Science Schools in parallel to leading her research team at the NCI-CCR.
Dr. Nussinov's 1978 paper proposed the dynamic programming algorithm for RNA secondary structure prediction. To date, this algorithm is still the leading method and is taught in bioinformatics classes in universities across Europe and in the U.S. The algorithm and its extension are described in SIAM J Appl Math, 35(1): 68-82, 1978 and Proc Natl Acad Sci U S A 77: 6309-13, 1980 (see also Biological Sequence Analysis: Probabilistic Models of Proteins and Nucleic Acids, Durbin R, Eddy SR, Krogh A, Mitchison G, eds., Cambridge University Press, 1998). Below are links to course lecture/reference materials.
Dr. Nussinov was also a pioneer in DNA sequence analysis (see Time Warps, String Edits, and Macromolecules: The Theory and Practice of Sequence Comparison, by David Sankoff and Joseph Kruskal, CSLI Press, 1999). In the early 1980s, she authored papers on the recurrence of nucleotide patterns, later becoming a trend in computational biology.
In 1990 Dr. Nussinov began to focus on proteins. In 1999, her NCI group proposed the model of 'conformational selection and population shift' as an alternative to ‘induced fit’ to explain molecular recognition. Biochemistry textbooks have championed the ‘induced fit’ mechanism for more than 50 years. The concept of conformational selection and population shift that her group introduced emphasized that all conformational states preexist, and that evolution has exploited them for function. This paradigm has impacted the scientific community's views and strategies in drug design, biomolecular engineering, and molecular evolution. Population shift is now broadly recognized as the origin of allostery, and thus signaling, across multimolecular complexes, pathways, and the entire cellular network. It also explains the effects of allosteric, disease-related mutations. The new concepts that we have contributed “have changed the way biophysicists and structural biologists think about protein folding, protein-protein interactions, and ligand binding” and is now included in a semester chemistry/biochemistry course in U.S. college so the students are “exposed to the depth and breadth of your work, which includes applications relevant to health such as cancer and inflammation, would be tremendously beneficial and inspiring to them”.
Her research team conducts studies of protein folding, binding and function. In particular, they investigate cancer-related proteins and pathways, aiming to figure out the mechanisms of signaling in cancer and inflammation pathways and oncogenic mutations. Dr. Nussinov’s research is interdisciplinary.
Dr. Nussinov is the author of more than 500 scientific papers and serves as editor-in-chief of PLoS Computational Biology and as an editor for the Journal of Biological Chemistry, Physical Biology, Proteins, BMC Bioinformatics, and other journals. Previously, she served two terms as editor for the Biophysical Journal. Dr. Nussinov is also a long-term member of the NIH Macromolecular Structure and Function D (MFSD) Study Section. She is a frequent speaker in colloquia and at international and domestic conferences and has given numerous invited talks at academic institutions around the world. She serves on site visit and grant review teams, and plays numerous roles in the scientific community.
Awards and Honors
In 2011, Dr. Nussinov was elected a Biophysical Society Fellow “for her extraordinary contributions to advances in computational biology on both nucleic acids and proteins.” She was also elected a Fellow of the International Society of Computational Biology (ISCB) in 2013; Elected Fellow of the American Physical Society (APS) “For extraordinary advancements in the understanding of the structure and function of biomacromolecules, an algorithm for predicting RNA secondary structure, and the Conformational Selection and Population Shift concept as an alternative to the Induced-Fit model in molecular recognition.” 2020; Elected Fellow, American Institute for Medical and Biological Engineering (AIMBE) College of Fellows (2021); A Festschript Special Issue in honor of Ruth Nussinov Achievements, ACS, Journal of Physical Chemistry, 2021. More in the CV.
Course Lecture/Reference Materials
Wikipedia: Nucleic Acid Structure
Wikiomics: RNA Secondary Structure Prediction
RNA Structure Determination (Center for Integrative Bioinformatics, Vrije Universiteit Amsterdam)
Job Vacancies
We have no open positions in our group at this time, please check back later.
To see all available positions at CCR, take a look at our Careers page. You can also subscribe to receive CCR's latest job and training opportunities in your inbox.