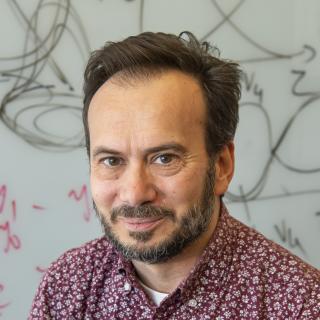
Grégoire Altan-Bonnet, Ph.D.
- Center for Cancer Research
- National Cancer Institute
- Building 37, Room 4134
- Bethesda, MD 20892
- 240-760-6888
- gregoire.altan-bonnet@nih.gov
RESEARCH SUMMARY
Our group's expertise is Systems Immunology: the ImmunoDynamics group (within the Laboratory of Integrative Cancer Immunology) has been developing experimentally validated quantitative models of different aspects of the immune system. In particular, we have addressed the interplay between the robustness and variability of self/non-self discrimination in the immune system. We are also focused on developing quantitative models of lymphocyte-lymphocyte communications via cytokine. Our current projects focus on the multicellular coordination of immune responses against tumors and pathogenic infections. We are particularly interested in applying machine learning tools and developing quantitative models of the integration of signal transduction, gene regulation, cytokine communications, cell differentiation, and proliferation/death across multiple spatio-temporal scales. Our long-term goal is to help in the development of tailored immunotherapies (e.g. against tumors).
Areas of Expertise
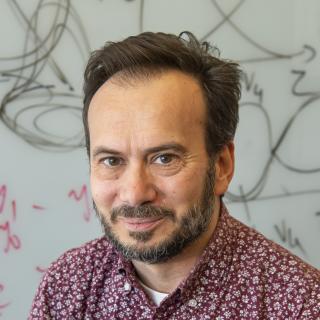
Grégoire Altan-Bonnet, Ph.D.
Research
We are interested in developing actionable models of the immune response to design and optimize new immunotherapies. Our premise is that the immune system is a self-organized collection of cells, whose individual activation must be harnessed, tuned, and coaxed at the population level. We rely on new quantitative methods (e.g., high-dimensional single cell analysis by spectral cytometry, robotics) and computational modeling to identify key limiting steps controlling the balance between response and tolerance in the immune system.
Our computational models are strongly interfaced with our experimental efforts. We model immunological events explicitly, starting from biochemical events (e.g., ligand/receptor interactions) to cellular (signal transduction and gene regulation) to population dynamics. Such integration of multiple spatial- and time-scales is leading to a better understanding of the dynamics of immune responses, and is opening new opportunities for external manipulation and immunotherapies. Our projects go from conceptual and fundamental aspects of the immune system, to very practical applications in the pre-clinical context (profiling of tumors, manipulation of immune responses against tumors).
Publications
- Bibliography Link
- View Dr. Altan-Bonnet's Complete Bibliography at Google Scholar
β-Coronaviruses Use Lysosomes for Egress Instead of the Biosynthetic Secretory Pathway
Stem-like CD8 T cells mediate response of adoptive cell immunotherapy against human cancer
CD3ζ ITAMs enable ligand discrimination and antagonism by inhibiting TCR signaling in response to low-affinity peptides
Engineering TCR-controlled Fuzzy Logic into CAR T-Cells Enhances Therapeutic Specificity
Biography
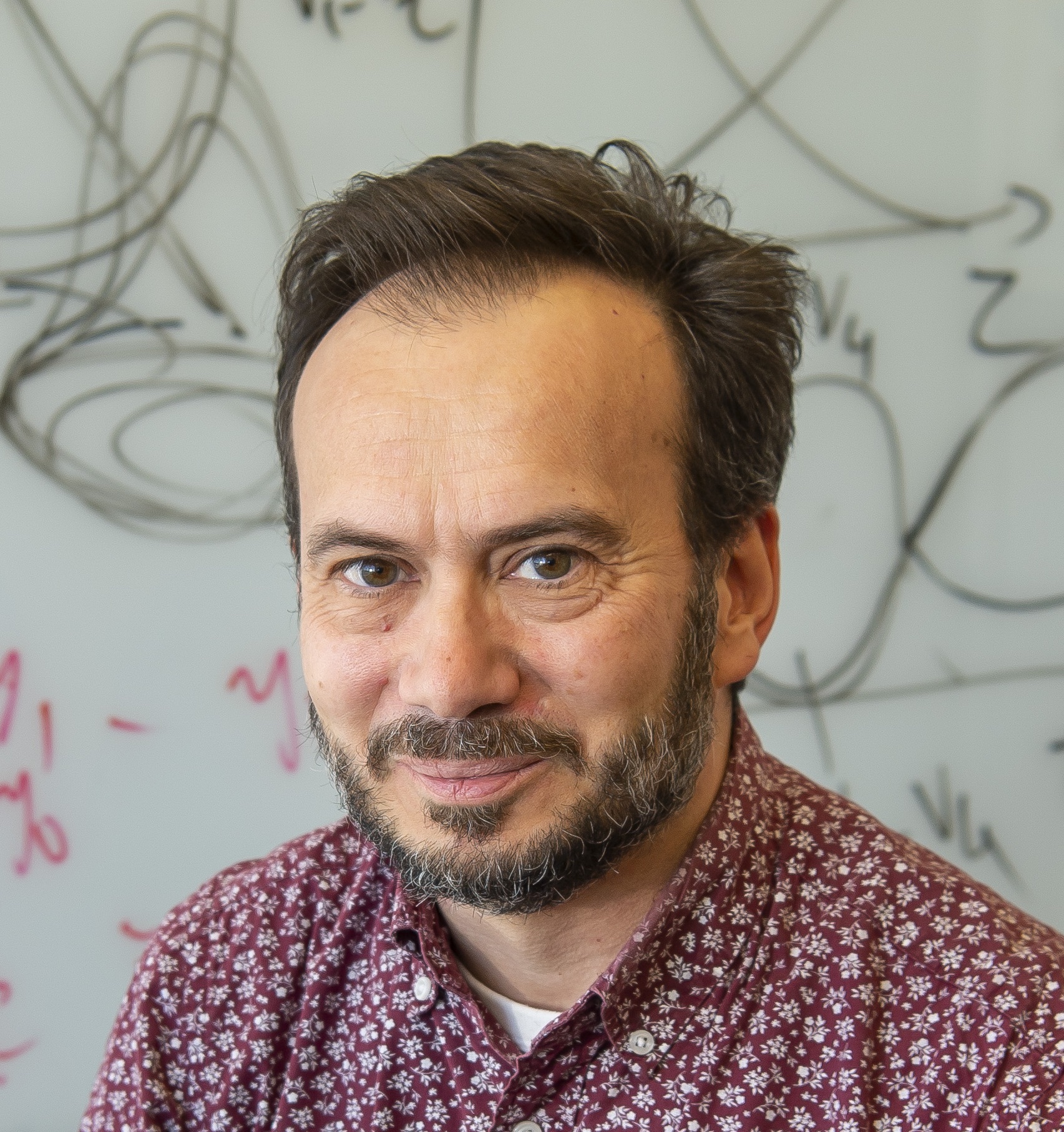
Grégoire Altan-Bonnet, Ph.D.
div>1992-1994 Bachelor of Science (Physics) - École Normale Supérieure (Paris, France)
1994-1995 Master of Science (Physics) - École Normale Supérieure (Lyon, France)
1995-2000 Ph.D. (Physics) - the Rockefeller University (New York, NY)
2000-2005 Research Fellow (post-doctoral studies) - Laboratory of Immunology, NIAID (Bethesda, MD)
2005-2011 Assistant member - Computational Biology & Immunology programs - Memorial Sloan Kettering (New York, NY)
2012-2015 Associate member - Computational Biology & Immunology programs - Memorial Sloan Kettering (New York, NY)
2016-2020 Earl Stadtman Investigator - Laboratory of Integrative Cancer Immunology (formerly, Cancer and Inflammation Program), Center for Cancer Research, NCI (Bethesda, MD)
Job Vacancies
We have no open positions in our group at this time, please check back later.
To see all available positions at CCR, take a look at our Careers page. You can also subscribe to receive CCR's latest job and training opportunities in your inbox.
Team
News
Projects
The Immunodynamics Group (led by Grégoire Altan-Bonnet) within the Laboratory of Integrative Cancer Immunology of the National Cancer Institute is dedicated to developing quantitative models of the immune system responding to tumors and infections. We contribute to the development of systems immunology by combining novel experimental approaches and computational/theoretical modeling. Here is our manifesto:
Top-to-bottom and bottom-up approaches for systems immunology.
In top-to-bottom approaches, we leverage the explosion of novel experimental techniques to monitor the multifactorial nature of immune responses: we use single-cell analyses (e.g. mass cytometry, spectral cytometry and single-cell transcriptomics) to characterize millions
of leukocytes, their phenotype, their state of activation and their overall contribution to the dynamics of immune responses
In our bottom-up approaches, we seek to build “simple” biochemically-accurate models of T cell activation to make targeted predictions about new functions for immune cells. This approach takes a commitment to reducing the immune complexity to few phenomenological equations, towards deriving generalizable understanding of immune responses.
In our top-to-bottom approach, we assemble large datasets (e.g. multiplexing donors, activation conditions, timepoints etc.) to explore the combinatorial complexity of immune responses. In that context, we use machine learning to derive new low-dimensional representation and biomarkers that best capture how immune cells function (e.g. identifying a specific cell types whose abundance predicts clinical outcomes goal is ).
Ultimately, we aim at merging both top-to-bottom and bottom-up approaches: 1) to learn a latent space that best captures the immune system; 2) to build models that explain the dynamics and functional relevance of this latent space.
Immunology and machine learning
The large datasets generated represent the biggest challenge and opportunity for the field of systems immunology. A typical experiment in the lab accumulates 100 Million cells, characterized by more than 40 single-cell features (e.g. up/down regulation of surface receptors, expression of transcription factors, activation of kinases, production of cytokines) across multiple activation conditions and time. We tackle these large high-dimensional datasets to derive a better understanding (i.e. a model) using tools from machine learning. In particular, auto-encoders and multi-layer perceptrons (MLP) have been used to build quantitative models of ligand discrimination by T cells. We are actively seeking novel tools from machine learning to improve our modeling capacities.
Of note, this interplay between systems immunology and artificial intelligence goes back-and-forth: while tools of machine learning obviously help tackle the combinatorial nature of our experimental observations, novel approaches in machine learning can also get inspired by the dynamic architecture of the immune system (this analogy was introduced by Paul François & Colleagues). At a fundamental level, we are exploring how the immune system must be conceptualized as a liquid brain, relying on the the multiscale functionalities of its leukocytes in order to classify pathological challenges and to decide accurate self/non-self discrimination.
Adaptive Kinetic Proofreading: a central scheme for decision making in the immune system.
How does the immune system discriminate between self tissues (that shall be left untouched) and non-self (that shall be attacked and cleared)? If viruses, bacteria and parasites clearly generate non-self antigens that trigger T cell activation, what about tumors? We have been building models of lymphocytes' decision making to understand how molecules (e.g. short peptides taken from virus, bacteria or tumors) determine functional response by immune cells. Our recent study on antigen encoding (re)discovered the "simple" scheme that accounts for T cells' sharp, specific, sensitive and rapid ligand discrimination. In a nutshell, the competition between a positive kinetic pruning of ligand/receptor activation and a negative feedback that limits the march towards activation is sufficient to recapitulate many aspects of T cell biology. In particular, our model predicts the existence of antagonism i.e. the nonlinear and often paradoxical response of T cells to mixture of ligands. In collaboration with Paul François (McGill University), we are committed to further explore the theoretical and practical underpinning of such adaptive kinetic proofreading scheme
Immune responses: coordinating cell activation from local to global effects
Collaborations:
Due to the obvious multidisciplinary nature of our research projects, we systematically seek novel collaborations to expand the range of experimental systems and theoretical approaches in our systems immunology projects. We document here few of the bridges we establish with other groups
We have a long-standing collaboration with Paul François’s group in the Department of Physics at McGill University to develop phenomenological and biochemically-accurate models of leukocyte activation. Our recent joint publication demonstrated the symbiotic nature of multiplexing dynamic measurements and machine learning to derive a compressed model of T cell activation and discrimination of ligands.
We collaborate with the DeVoe lab in the Department of Mechanical Engineering of the University of Maryland @ College Park to develop novel microfluidic devices to assemble, monitor and manipulate immune reactions.
The laboratories of the National Institutes of Health in Bethesda are extremely collaborative, allowing the deployment of our systems immunology/machine learning toolbox to many projects, including:
- Innate responses against viral infections (with Nihal Altan-Bonnet’s group at NHLBI)
- T cell development and signaling (with Paul Love’s group at NICHD)
- Cytokine signaling (with Carmen Molina Paris & Grant Lythe at the university of Leeds and Anton Zilman’s group at the university of Toronto)
- Cytokine-mediated regulations of self/non-self discrimination (with Ron Germain’s group at NIAID) and responses to tumors (with Nadège Bercovici’s group at Institut Gustave Roussy and Audrey Gérard’s group at Oxford University).
Finally, we have established collaborations with clinical labs to realize the promises of bench-to-bedside (and back!). Of note, we have a strong collaboration with Nirali Shah’s and Naomi Taylor’s groups within the Pediatric Oncology Branch at NCI to characterize, model and optimize Chimeric-Antigen Receptor (CAR) T cell therapies. We apply our robotic/machine learning pipeline to characterize CAR-T cells from clinical products as well mouse models. We aim at better understanding how CAR-T not only kill tumor targets, but also differentiate, proliferate and persist towards sustained eradication of tumors. This collaborative project with the Shah/Taylor groups has been supported by an intramural synergy grant of the National Cancer Institute.
Covers
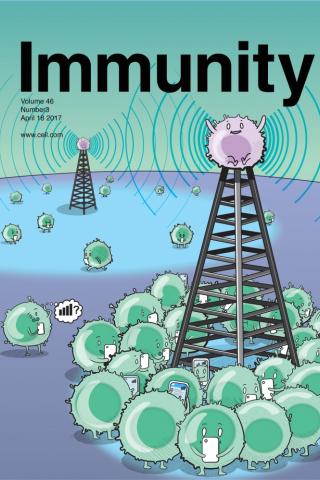
A Tunable Diffusion-Consumption Mechanism of Cytokine Propagation Enables Plasticity in Cell-to-Cell Communication in the Immune System
Immune cells communicate by exchanging cytokines to achieve a context-appropriate response, but the distances over which such communication happens are not known. Here, we used theoretical considerations and experimental models of immune responses in vitro and in vivo to quantify the spatial extent of cytokine communications in dense tissues. We established that competition between cytokine diffusion and consumption generated spatial niches of high cytokine concentrations with sharp boundaries. The size of these self-assembled niches scaled with the density of cytokine-consuming cells, a parameter that gets tuned during immune responses. In vivo, we measured interactions on length scales of 80–120 mm, which resulted in a high degree of cell-to-cell variance in cytokine exposure. Such heterogeneous distributions of cytokines were a source of non-genetic cell-to-cell variability that is often overlooked in single-cell studies. Our findings thus provide a basis for understanding variability in the patterning of immune responses by diffusible factors.
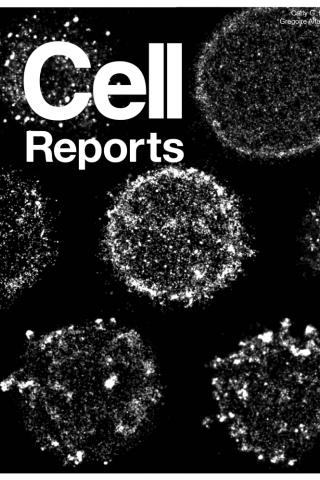
Constitutive Activation of the B Cell Receptor Underlies Dysfunctional Signaling in Chronic Lymphocytic Leukemia
In cancer biology, functional interpretation of genomic alterations is critical to achieve the promise of genomic profiling in the clinic. For Chronic Lymphocytic Leukemia (CLL), a heterogeneous disease of B-lymphocytes maturing under constitutive B-cell receptor (BCR) stimulation, the functional role of diverse clonal mutations remains largely unknown. Here, we demonstrate that alterations in BCR signaling dynamics underlie the progression of B cells toward malignancy. We reveal emergent dynamic features, namely bimodality, hypersensitivity, and hysteresis, in the BCR signaling pathway of primary CLL B cells. These signaling abnormalities in CLL quantitatively derive from BCR clustering and constitutive signaling with positive feedback reinforcement, as demonstrated through single-cell analysis of phosphor-responses, computational modeling, and super-resolution imaging. Such dysregulated signaling segregates CLL patients by disease severity and clinical presentation. These findings provide a quantitative framework and methodology to assess complex and heterogeneous leukemia pathology and to inform therapeutic strategies in parallel to genomic profiling.
https://papers.ssrn.com/sol3/papers.cfm?abstract_id=3346981
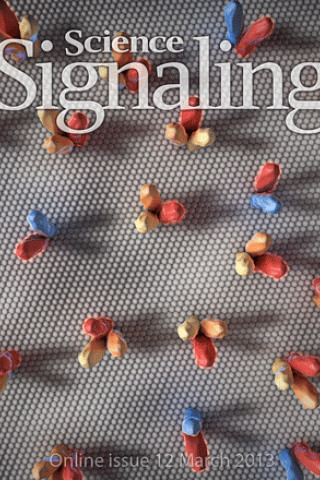
Cell-to-Cell Variability Analysis Dissects the Plasticity of Signaling of Common γ Chain Cytokines in T Cells
This Research Article presents flow cytometric and mathematical analyses of the effect of increasing the abundance of the α subunit of the interleukin-2 (IL-2) receptor (IL-2Rα) on the responsiveness of mouse T cells to cytokines of the γc family, which includes IL-2 and IL-7. The data suggest that the increased IL-2Rα abundance decreases the sensitivity of T cells to IL-7 by sequestering the γc subunit away from the IL-7R, rendering it nonfunctional. The image shows an artist's depiction, looking down onto the cell surface, of the differential abundance of functional IL-7Rs (dimers) and IL-2Rs (trimers), both of which contain the γcsubunit (red), as a consequence of increased IL-2Rα abundance. [Image: Chris Bickel/AAAS]
https://www.ncbi.nlm.nih.gov/pubmed/23482665