Oncogenomics Section
Javed Khan, M.D.
Research
Mission of the Oncogenomics Section
The mission of the Oncogenomics Section is to harness the power of high throughput genomic and proteomic methods to improve the outcome of children with high-risk metastatic, refractory and recurrent cancers. The research goals are to integrate the data, decipher the biology of these cancers and to identify and validate biomarkers and novel therapeutic targets and to rapidly translate our findings to the clinic.
1. Comprehensive Omics Analysis: a) Applying high-throughput genomics, proteomics mathematical modeling and bioinformatics to characterize currently incurable malignancies including metastatic, resistant and relapsed tumors for the identification and validation of biomarkers and therapeutic targets. b) Genome wide mutational screening of germ-line DNA.
2. Targeted Therapeutics: a) High-throughput CRISPR knock out, CRISPRi and CRISPRa, small molecule, natural products, and drug screening for high-risk pediatric malignancies. b) Development of precision therapies against existing and newly identified targets.
3. Translational Oncology: Leverage the existing clinical and scientific strengths within the CCR including phase 1/2 therapeutics, immune/vaccine therapy and molecular biology to translate these findings to the clinic in an environment where there is state-of-the-art clinical care.
Team
Job Vacancies
We have no open positions in our group at this time, please check back later.
To see all available positions at CCR, take a look at our Careers page. You can also subscribe to receive CCR's latest job and training opportunities in your inbox.
Covers
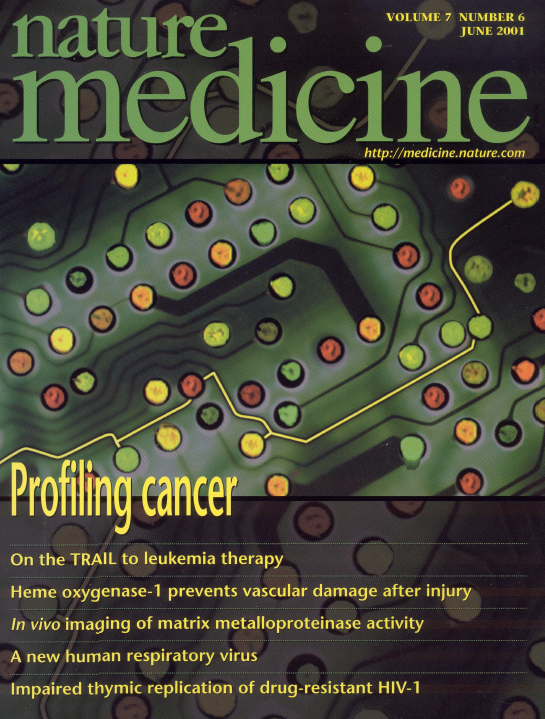
Classification and diagnostic prediction of cancers using gene expression profiling and artificial neural networks
Abstract
The purpose of this study was to develop a method of classifying cancers to specific diagnostic categories based on their gene expression signatures using artificial neural networks (ANNs). We trained the ANNs using the small, round blue-cell tumors (SRBCTs) as a model. These cancers belong to four distinct diagnostic categories and often present diagnostic dilemmas in clinical practice. The ANNs correctly classified all samples and identified the genes most relevant to the classification. Expression of several of these genes has been reported in SRBCTs, but most have not been associated with these cancers. To test the ability of the trained ANN models to recognize SRBCTs, we analyzed additional blinded samples that were not previously used for the training procedure, and correctly classified them in all cases. This study demonstrates the potential applications of these methods for tumor diagnosis and the identification of candidate targets for therapy.
Classification and diagnostic prediction of cancers using gene expression profiling and artificial neural networks. Khan J, Wei JS, Ringnér M, Saal LH, Ladanyi M, Westermann F, Berthold F, Schwab M, Antonescu CR, Peterson C, Meltzer PS. Nat Med. 2001 Jun;7(6):673-9.
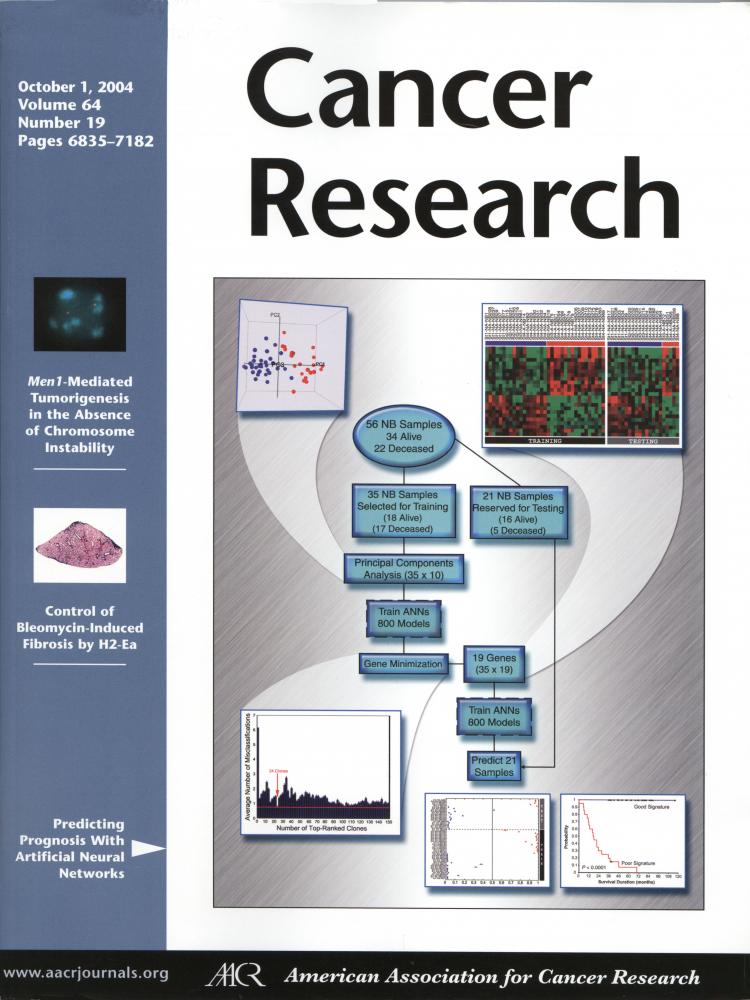
Prediction of clinical outcome using gene expression profiling and artificial neural networks for patients with neuroblastoma
Abstract
Currently, patients with neuroblastoma are classified into risk groups (e.g., according to the Children’s Oncology Group risk-stratification) to guide physicians in the choice of the most appropriate therapy. Despite this careful stratification, the survival rate for patients with high-risk neuroblastoma remains <30%, and it is not possible to predict which of these high-risk patients will survive or succumb to the disease. Therefore, we have performed gene expression profiling using cDNA microarrays containing 42,578 clones and used artificial neural networks to develop an accurate predictor of survival for each individual patient with neuroblastoma. Using principal component analysis we found that neuroblastoma tumors exhibited inherent prognostic specific gene expression profiles. Subsequent artificial neural network-based prognosis prediction using expression levels of all 37,920 good-quality clones achieved 88% accuracy. Moreover, using an artificial neural network-based gene minimization strategy in a separate analysis we identified 19 genes, including 2 prognostic markers reported previously, MYCN and CD44, which correctly predicted outcome for 98% of these patients. In addition, these 19 predictor genes were able to additionally partition Children’s Oncology Group-stratified high-risk patients into two subgroups according to their survival status (P 0.0005). Our findings provide evidence of a gene expression signature that can predict prognosis independent of currently known risk factors and could assist physicians in the individual management of patients with high-risk neuroblastoma.
Prediction of clinical outcome using gene expression profiling and artificial neural networks for patients with neuroblastoma. Wei JS, Greer BT, Westermann F, Steinberg SM, Son CG, Chen QR, Whiteford CC, Bilke S, Krasnoselsky AL, Cenacchi N, Catchpoole D, Berthold F, Schwab M, and Khan J. Cancer Res. 2004 Oct 1;64(19):6883-91.