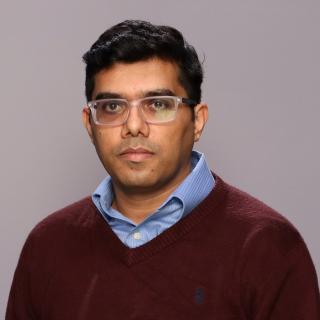
Ayush Raman, Ph.D.
- Center for Cancer Research
- National Cancer Institute
- Building 41, Room B620
- Bethesda, MD 20892
- 240-858-7805
- ayush.raman@nih.gov
RESEARCH SUMMARY
Ayush Raman is a staff scientist working with the labs of Shalini Oberdoerffer and Daniel Larson at the Laboratory of Receptor Biology and Gene Expression at the National Cancer Institute. Prior to his current position, he was a Postdoctoral Associate at Broad Institute and received his PhD from Baylor College of Medicine.
For his doctoral thesis, he pursued his research on two major themes: a) using integrative genomics and systems biology methods to analyze large-scale multi-omics sequencing data and b) developing statistical methods/approaches in identifying the latent variables, also known as batch effects and sources of spurious results such as failure to measure baseline variability in RNA-seq datasets. For his postdoctoral training, he built on his previous training in transcriptional regulation by aiding in the development of multi-omic single-cell assays for understanding the role of DNA methylation in the development, senescence and diseases.
Areas of Expertise
Biography
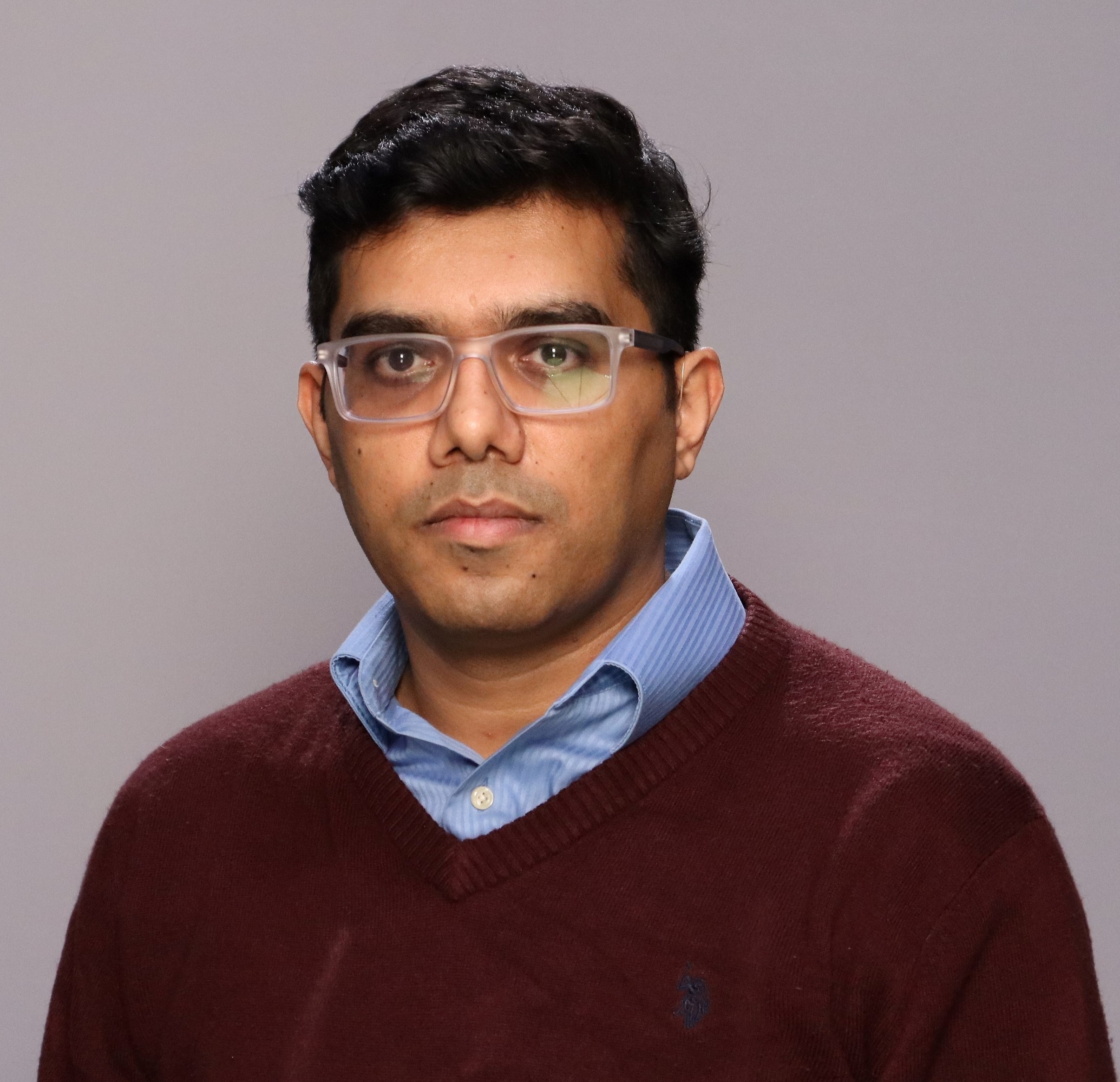
Ayush Raman, Ph.D.
Dr. Ayush Raman obtained his PhD in Quantitative and Computational Biosciences from Baylor College of Medicine, where he worked with Prof. Zhandong Liu and Prof. Huda Zoghbi on batch effects in next-generation sequencing datasets, reproducibility & meta-research, and also worked with Prof. Kunal Rai in the field of the cancer epigenomics. He then moved to Broad Institute, where he worked with Prof. Martin Aryee and Prof. Alex Meissner to understand the dynamics of gene regulation using single-cell genomics.
Prior to his Ph.D. studies, he worked as a Bioinformatics Scientist in the lab of late Prof. Ilya Shmulevich at Institute for Systems Biology, Seattle. He received his Master’s degree in Computational Biology from Carnegie Mellon and did his undergraduate studies at Vellore Institute of Technology, where he earned an Engineering degree in Bioinformatics.
Dr. Raman comes with a data-driven and disease-agnostic approach, where he uses his skillsets in machine learning and statistical analysis to a) understand and infer inherent biases due to the technology and non-biological signals or noise in the dataset, b) generate & test hypotheses by modeling publicly available datasets, and c) use computational methods for integration of multi-modal high throughput sequencing datasets, including bulk and single-cell RNA-seq, ChIP-seq, ATAC-seq and DNA methylation. He has expertise in the analysis of other datasets, such as CRISPR-based assays (CRISPRi, CRISPRa and dual assays) and long-read sequencing datasets.
In addition, he develops tools, approaches and pipelines for genomic data analysis and has extensive experience working with publicly available datasets, including GEO, TCGA, CCLE, GTex, Gene Expression Omnibus and DepMap.